The Ö÷²¥´óÐã is famous for high-quality content, stunning visuals and breath-taking pictures. The visual data analytics group in Ö÷²¥´óÐã Research & Development is creating new and efficient artificial intelligence (AI) engines to support high-quality content creation and delivery as well as the responsible use of AI.
Project from 2020 - present
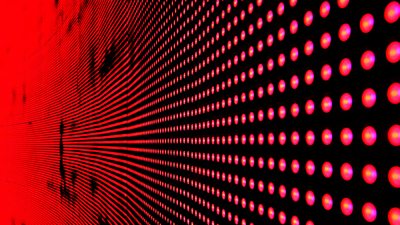
Why are we doing this?
Artificial intelligence is transforming the ways information reaches us through means such as recommendations and delivery. It also influences how content is created. For example, AI and in particular, machine learning (ML) can be used to summarise videos, enhance picture quality and process content. This has the potential to have a significant influence on various aspects of people's lives, making those working with AI and ML responsible for its use. Therefore, our goal at Ö÷²¥´óÐã R&D is to put technology advances to exciting new uses, which deliver new, better content for the Ö÷²¥´óÐã audiences. Simultaneously we are working to make technology based on ML more predictable and trustworthy (i.e. have mechanisms to help us understand what a machine has learned, and make sure we know the results are reliable). More specifically, we are researching how to efficiently and ethically use the power of AI applied to images and videos, some of the richest and most complex forms of data.
What we're doing
Applying ML to the creation, enhancement, classification and compression of multimedia content is . Notably, we have successfully demonstrated how AI can support innovation in the production and distribution of video. Examples include the enhancement of poor quality video, such as user-generated content and archive material, to provide new high-quality footage. Within our COGNITUS system, deep learning is applied to increase resolution, evaluate the quality and automatically enrich the video with metadata. Large amounts of user-generated content can be quickly and easily made available to programme-makers using . We are also making progress using social media data to edit visual stories automatically. and place them in a coherent, sequential manner to report on an event.
Machine Learning and Video Compression
We can also address the limitations of delivery channels by improving content compression with machine learning. We are working on the next generation of compression standards to enable a significant reduction in the bandwidth needed to deliver high-definition video. Since this typically comes with increased computational complexity, we are looking at how to use ML more efficiently – either in its simple forms as decision trees or more advanced .
Deep Learning to Colourise Historical Content
Historical content can be restored more cheaply using AI techniques. For example, adding colour to black and white video has been an expensive and time-consuming task - until now. Recent advances with deep learning have enabled the development of new colourisation algorithms based on deep learning. Ö÷²¥´óÐã R&D has recently developed a new method to perform the job more efficiently, based on Generative Adversarial Networks (GANs). GANs are a type of neural network used to create new data from an existing dataset (in this case images). In particular, using knowledge from a given set of colourised content. This enables us to obtain more natural, realistic and plausible colourful content.
Interpretability of AI
In future, the use of AI will open up new challenges and opportunities, such as the interpretability and explainability of AI, a reduction of the cost of AI training and deployment, and learning from a small amount of data. Some of these challenges arise because although the inputs and outputs are known, there is little transparency on how these methods arrive at their predictions - network models are commonly applied in a black-box manner. We, therefore, work on specific aspects of understanding these inner processes, which allows us not only to explain and verify the outputs of AI models but also to address complexity cost and data shortage. For example, neural networks can improve selected stages of the video compression process, and by understanding this, we can remove redundancies (steps that are not critical). This further enhances video compression performance to enable the delivery of high-quality video at efficient speeds.
Collaborations
On this journey, we are not alone. In addition to working with colleagues across the Ö÷²¥´óÐã, we are training new researchers and achieving significant research results in collaboration with the H2020 Marie-SkÅ‚odowska-Curie European Training Network and in partnership with top academic institutions in the UK through .